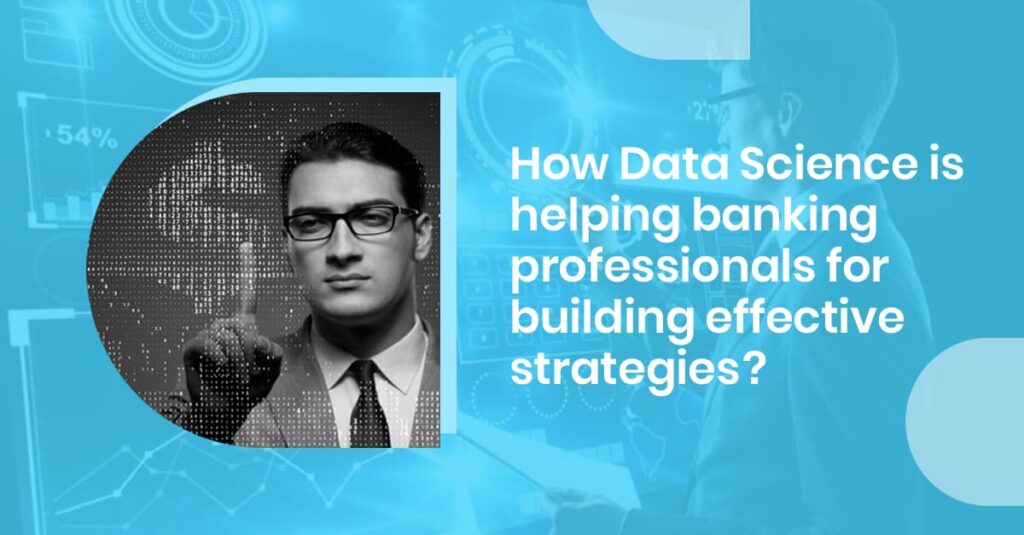
How data science is useful for banking professionals?
Data science in the banking industry is causing a sweeping transformation as per the changing consumer behaviours and expectations, more strict regulatory guidelines and highly competitive environment bind a potential success in the area of banking.
In Standard Chartered, Data Scientists tend to convert raw data to useful insights into business decision-making. This is achieved by leveraging tools and techniques, such as Machine Learning, Qlikview for visualizations, Hadoop for handling Big Data, R & Python programming for building data models, etc. Banks have always been a custodian of customer data, but they lack the technological and analytical capability to derive value from the data.
Data science will be the linchpin of future personalised banking services, Bank of Ireland’s “Colin Kane”. Data science is already a core fixture at Bank of Ireland, where various teams of data scientists and data experts are involved in analytics functions such as risk analytics, anti-money laundering (AML) fraud, marketing and customer experience.
The analytics market in India is growing in leaps and bounds. “The analytics business in India has created progress in terms of adoption across industries, nonetheless it’s obscurity on the brink of its potential being a broach, applying analytics to banking and finance is crucial, profitable, and intensely appreciated, each for organizations and professionals. That Decades went when a typical bank client would walk into a bank and be greeted by a banker who knew his name, his personal backgrounds and the way best to serve his personal banking desires.
This can be quite a recent model wherever banks have non-inheritable and preserved the customer’s trust and served them for a protracted time. However, things modified. individuals usually are engaged in multiple assignments and travels to completely different geographical locations. If at some point he stays in the national capital, the immediate next day he might get to visit Paris on his business assignments. In such conditions, it’s difficult for a banker to trace his personal preferences and whereabouts to satisfy his desires. Massive knowledge offers insight into several complicated areas of individual’s life together with their manner, needs, and preferences of their customers so it’s simple for banks to personalise services to the requirements of every individual.
For a protracted time, the banks miserably didn’t utilize the data generated by their own business. the massive knowledge has become a game-changer in remodelling their business method and conducts to spot business opportunities and potential threats. Generally, banks and money establishments notice massive knowledge from sources like knowledge, transactions, helplines, emails, social media, external feeds, support, audio, video and a few alternative sources.
Data, as we know, has been the driving issue for several sectors and organizations. And one sector wherever it adds extreme worth is that the one that deals with capital – banking and finance. Finance features is a crucial role in boosting the worth and growth of a business and banking permits quality and wealth generation. As key business and economic functions, banking and finance overlap with analytics as they inherently cope with the information.
Applying Analytics to money information opens whole new avenues of insights and understanding of the business, the market, their performance, and their growth. The ongoing transformation is opening doors to several opportunities. Banks must ensure that they can cost-effectively acquire new customers while retaining existing ones. And to expand their reach and profitability, they must also tighten their focus on the expanding digital world. Analytics, big data and data science can unlock a world of new possibilities.
With the proper use of data science, banks can better understand prospect/customer relationships by exploring ever-changing transactional and interactional behaviours. New digital marketing technologies, such as Web sites, e-mail, mobile apps and social networks, are helping banks better target their customers and improve engagement.
Moreover, advanced segmentation strategies are helping them boost their marketing effectiveness by identifying niches based on consumer behaviour. Many banks are just beginning to consolidate and utilize the internal data elements at their disposal, such as debit and credit transactions, purchase histories, channel usage, communication preferences, loyalty behaviour, etc. And when it comes to big data, banks have collected large amounts of information from a variety of sources, such as transaction details and spending behaviours. The addition of newer sources, including Web server logs, Internet clickstreams, social media activity and mobile-phone call details, has opened the floodgates on the data sets that can be mined for meaning.
Introduction of massive knowledge in banking has destroyed several ground rules of business and remodelling the landscape of the money services business. With an enormous volume of information gushing from innumerable transactions, the banks try to seek out innovative business concepts and risk management solutions.
Every set of data gathered over an amount and tell a novel story and show the post for a particular future amount so a house will take advantage of this information to realize a competitive edge up the market. This massive analytics knowledge will improve the extrapolative power of risk models employed by banks and money establishments, analytics also can be employed in credit management to find fraud signals and same will be analyzed in real-time victimization AI. On a significant note, banking and finance business cannot understand knowledge analytics
in isolation.
Alongside distinguishing business opportunities, they must establish security threats, the prevalence of fraud and potential remedies. Further, they must arrange to connect analytical knowledge across division and structure silos. In several of the normal banking entities in India haven’t nonetheless begun their massive knowledge activities. The banks have a footing in capitalizing on those opportunities.
Therefore, massive knowledge science not solely brings new insights to the banks, however, it conjointly allows them to remain a step previous the sport with advanced technologies and analytical tools. As an early warning system, data science solutions can help banks quickly identify potentially fraudulent behaviour before the fraud becomes material. For example, individual cardholders are creatures of habit.
Cardholders have “favourites “ or recurrences over a wide variety of objects in their transaction streams. These objects might include favourite ATMs that are close to work or home or gas stations along a daily commute, as well as preferred grocery stores and online sites for shopping. An analytics technique that could be used to improve fraud management is to identify cardholder favourites, in order to distinguish between “in-pattern,” or normal, customer spending and “out-of-pattern” suspicious transaction activity.
This enables faster fraud detection at much lower false-positive rates (declines on legitimate transactions). Text analytics of unstructured data can help banks identify patterns of information that indicate the likelihood of fraud. Text mining of insurance claim descriptions (written and recorded) provided by bogus claimants uncovered some very interesting facts. It turns out that certain phraseologies (the use of “ed” rather than “ing” on the end of verbs, for instance), are extremely indicative of
fraudulent claims. This is due to the different ways in which people rely on stories they actually experienced vs. those they concocted; for instance “I
was walking” is indicative of someone recounting an actual experience whereas “I walked” often turns out to be indicative of someone describing
a fictitious event.
DBS Bank, Southeast Asia’s largest bank by market capitalisation, is driving a digital transformation process with data at its heart, with goals including the growth of its customer base in India from 1.8 million to 5 million by 2021. DBS will look into improving its data analytics set up to enhance areas like robotic process automation – 8 per cent of customer interactions are handled by bots – and other more advanced applications such as anti
money laundering: ït’s still early days but we will be enhancing analytics to drive a lot more productivity and more added-value services and processes to the bank.
For instance, machine learning can be used to understand customer sentiments.
All calls to the bank’s call centres are recorded. They can be converted to text and then machine learning algorithms can be used on the analytics platform to understand the sentiment. Problems can be flagged so that the bank can reach out to the customers.
Ultimately behavioural information and machine learning, in combination with biometrics could even enable ‘invisible authentication’, where a customer no longer needs to provide any supporting documents or use a physical device for transactions According to Gartner report, Yes Bank carried out the successful deployment of data analytics for enhancing its business impact, integrating data science in their core model showcasing the urge to create data science-driven decision making.
Techniques to gain insights into customer behaviour, services and predictive models using different types of customer transactional and behavioural data. Impacting the revenue growth with high margins, controlling risk management and enhancing customer experience. Axis, third-largest private-sector banks in India, recently launched a hackathon to solve a signature recognition model.